Unveiling the Best Choice: AWS Bedrock vs. AWS SageMaker – Which is the Ultimate Solution for Your Business?
- chaitanyagaajula
- Jan 1
- 4 min read
In the rapidly changing world of artificial intelligence and machine learning, choosing the right platform can be overwhelming. Amazon Web Services (AWS) provides prominent solutions through AWS Bedrock and AWS SageMaker, each built to support distinct machine learning applications. So how does a business decide? This guide will explore the unique features of both services, highlight their appropriate use cases, and help organizations make clear, informed choices.
Understanding AWS Bedrock
AWS Bedrock represents a significant advancement in AWS’s services, allowing developers and businesses to easily build and scale generative AI applications. It is designed to help users implement machine learning models built on pre-trained foundational models sourced from various AI providers.

AWS Bedrock suits companies looking to infuse generative AI into their applications with minimal upfront investment for training. For instance, a retail business could utilize Bedrock's tools to create customized product recommendations, enhancing customer experience without the need for extensive model training resources.
This service offers flexibility in model selection and robust fine-tuning capabilities, allowing businesses to adapt their AI solutions as needs evolve. A strong focus on security and compliance ensures organizations can protect sensitive data while leveraging these technologies.
Understanding AWS SageMaker
Conversely, AWS SageMaker is a comprehensive machine learning platform that empowers developers and data scientists to build, train, and deploy machine learning models effectively. Known for its extensive toolset, SageMaker simplifies the end-to-end machine learning process—from preparing data to training models and finally deploying them.
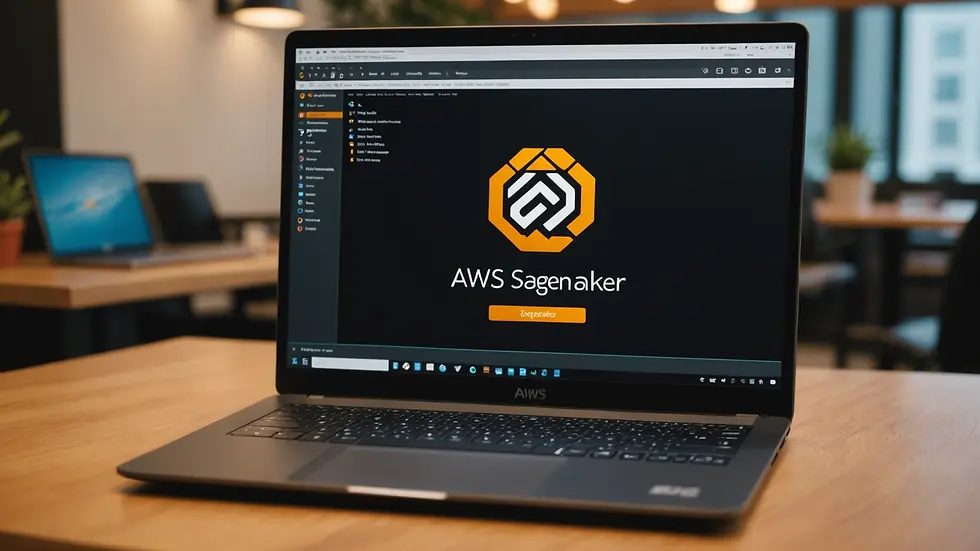
One of SageMaker’s key strengths is its automation. It can automate the machine learning lifecycle using built-in algorithms, which significantly reduces the time needed for setup. For example, a financial institution could use SageMaker’s features to automate fraud detection through tailored algorithms, improving both efficiency and accuracy in operations.
Moreover, SageMaker integrates seamlessly with various data sources and offers capabilities like SageMaker Autopilot. This feature helps users automate model creation while providing clear insights into performance, reducing the skill barrier for businesses eager to engage in machine learning.
When to Choose AWS Bedrock
Choosing AWS Bedrock may be beneficial in the following scenarios:
Generative AI Focus: If your primary goal is to leverage generative AI technologies, Bedrock gives you immediate access to advanced foundational models.
Speed of Deployment: Organizations seeking fast deployment of AI applications without the burdensome process of training custom models will find Bedrock's pre-built solutions valuable.
Experimentation with Minimal Overheads: Smaller enterprises or startups can benefit from Bedrock's reduced operational costs while still accessing strong AI capabilities.
Diverse Model Selection: Companies that want to experiment with a variety of models from different AI providers may find Bedrock's structure advantageous.
When to Choose AWS SageMaker
On the other hand, AWS SageMaker may be the preferred choice for:
Custom Model Development: Businesses in need of tailored solutions for unique challenges will find SageMaker's extensive customization options invaluable.
Advanced Data Science Workflows: Data scientists looking for comprehensive tools, including data integration and automated configuration, might favor SageMaker's capabilities for complex projects.
Robust Deployment Options: If your organization requires different deployment methods—like real-time analytics or batch processing—SageMaker can accommodate these varied needs.
Resource Optimization: Companies capable of managing their infrastructure can utilize SageMaker’s automation features to optimize resource use while driving effective model performance.
Cost Implications
Cost considerations play a crucial role in selecting between AWS Bedrock and AWS SageMaker. Bedrock typically follows a straightforward pricing model based on usage, making it appealing for organizations focusing on generative applications. For example, businesses using Bedrock for a marketing campaign may pay only for the resources they consume during the campaign.
Conversely, SageMaker provides a more detailed pricing structure, allowing for cost-saving measures such as utilizing spot instances. Organizations can optimize their expenditures based on workload demand, leading to a potentially lower overall investment if managed correctly.
Given these financial factors, assessing your organization's budget and long-term AI strategy is critical.
The Integration of Both Services
Some organizations might find it valuable to utilize both AWS Bedrock and AWS SageMaker. For instance, a startup could begin with Bedrock's ready-to-use models for initial AI projects and later switch to SageMaker as their needs evolve and custom solutions become necessary.
This hybrid strategy allows businesses to remain flexible while maximizing the strengths of each service, adapting as their requirements grow.
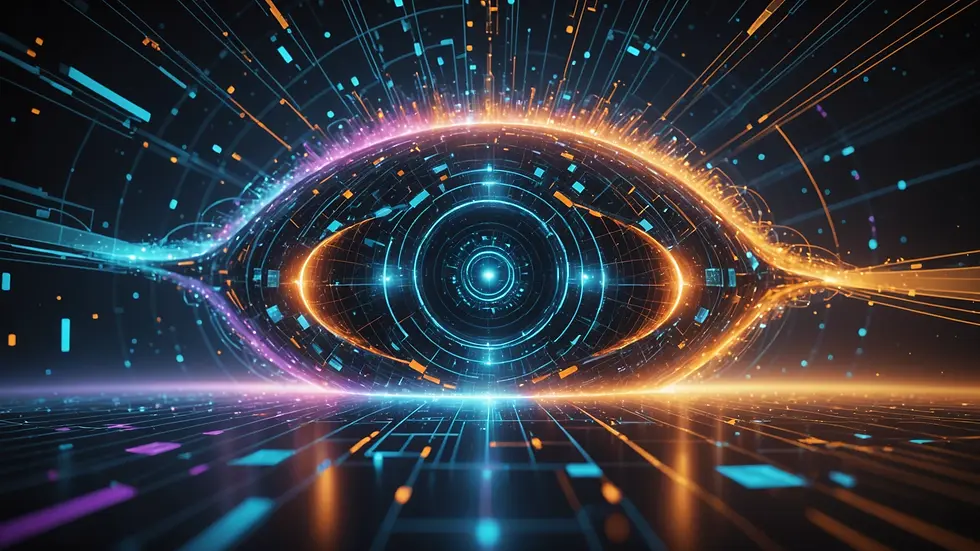
Future Considerations
Considering future growth and tech trends is essential when choosing between AWS Bedrock and AWS SageMaker. The landscape of artificial intelligence is always evolving. Both services will likely introduce new features and capabilities, enhancing their value to users.
When making your decision, evaluating the ability to scale your AI solutions while keeping control over your models will significantly influence your choice.
Making the Right Choice
Deciding between AWS Bedrock and AWS SageMaker is more than just a technological choice; it reflects your organization’s broader strategy toward AI integration. By understanding the unique features and ideal use cases of each service, businesses can align their decisions with their operational needs and long-term goals.
Whether you aim for fast deployment of generative AI applications with AWS Bedrock or desire to fine-tune machine learning models through AWS SageMaker, the best choice rests on your specific requirements and objectives.
Taking the time to analyze your organization’s needs and the strengths of each service is an essential step toward unlocking the full potential of artificial intelligence and machine learning in your business operations.
Comments